Due to the existence of big data, the use of benchmarks attained from information is gaining popularity in today’s society. Two terms that pop out in such discourses are data analysis and data analytics Even as there is likely to be a considerable overlap in the denomination of the two, they essentially denote two different concepts, and the disparity between them is one of kind rather than degree. While there are some distinct differences between data analysis and data analytics which we shall explore in this post, the key reason why it is important to differentiate between the two is to gain a better insight of the two when it comes to your business.
Definition and Scope:
Data Analysis: This is the process of assessing, scrubbing, reshaping, and visualizing the data with the aim of finding useful insights, drawing conclusions, and decision making. It is about studying facts with the aim of knowing what has taken place in the past and the causes that led to such incidents. Descriptive analytics is more real-time and presents questions such as, “What was the revenue generation in the last quarter?” or “Why did customer interaction reduce in March?
Data Analytics: Data analytics, however, goes further than data analysis, though this is part of data analytics. It is a branch of analytics that goes beyond simple data analysis and applies sophisticated methods such as predictive modeling, statistical analysis and machine learning to not only explain the past but also anticipate the future behaviors. It is the process of looking for insights from data to answer questions such as ‘How many sales will we make next quarter?’ or ‘Which of our customers are likely to cancel in the next six months?’
Tools and Techniques:
Data Analysis: The tools of data analysis are in many ways less complex and may include spreadsheets such as Excel or Google Sheets, statistical packages such as SPSS or SAS, and data visualization tools such as Tableau or Power BI. This would involve the use of other methods such as descriptive statistics, correlations, or simple regression.
Data Analytics: Data analysis calls for even higher levels of tools and methods most of the time. These could be general-purpose languages such as Python or R for statistical analysis and data modeling, large data processing frameworks like Hadoop or Spark and machine learning frameworks such as scikit-learn or TensorFlow. Such approaches may include regression analysis, decision trees, time series analysis or natural language processing.
Focus and Application:
Data Analysis: It is mainly an historical analysis as far as the type of data is concerned. It is more about that which has already taken place. This is useful when doing financial statements, sales forecasts or even assessing the success of a marketing blitz after the exercise.
Data Analytics: It does not stop at the transgression itself but moves onto the present and even future. They involve anticipating certain events and suggesting what actions should be taken. That is vital for such use cases as, for instance, customer profiling (who would react better to which product), sales prediction, or stock exchange prediction in finance.
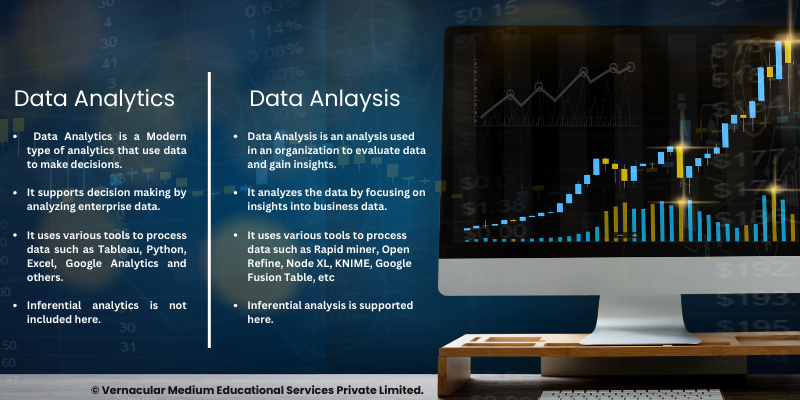
Role in Decision Making:
Data Analysis: Gives information that helps in decision making especially in the strategic, which are usually short-term. For instance, a data analyst might use the results of his or her analysis to discover that product A performs better than product B on the weekends. This insight could lead to a decision to promote product A more heavily on weekends since it is more likely to be bought then.
Data Analytics: ELT has been found to be used to provide information on strategic, and sometimes even extended, managerial decisions. For instance, a data analytics model is likely to forecast a decline in demand for a given product line for the next five years due to shifting consumer trends. It could culminate in a strategic move that involves the management deciding to discontinue that line of products and instead venture into a new line of products.
Skills and Roles:
Data Analysis: Basically, a data analyst requires good knowledge of statistics, ability to create good visualizations, and understanding the domain. They should be able to calculate, develop meaningful numbers and graphical representations and also appreciate the business implications of such figures.
Data Analytics: A data scientist or a data analytics expert should possess all data analyst skill sets but in addition, should be proficient in statistics, machine learning, and possibly programming. It is vital for them to be able to construct sophisticated models, understand what these models are telling them, and incorporate these models into business environments.
Conclusion:
Therefore, it can be concluded that data analysis and data analytics are interconnected concepts, as well as two different approaches to manage data for decision making, with different objectives, strategies, methods, and cases of use. Data analysis is something that is more restricted to understanding the past, whereas data analytics is the extension of the same in the future.
In practice, most data-driven organizations require both types of data to be effective and efficient in their operations. They employ the use of data analysis on their current status and data analytics for the future evolution. At least if we understand the strengths of each, it means that we are applying the appropriate strategy to each business question, which is a more effective way of reaching a conclusion.
**********
Disclaimer:- Views expressed are the author’s own.
Beautiful article
Thank you madam.😊